15 Jul 2024
Revolutionizing Dental Implants: How Deep Learning is Transforming 3D Bone Segmentation and Tooth Prediction
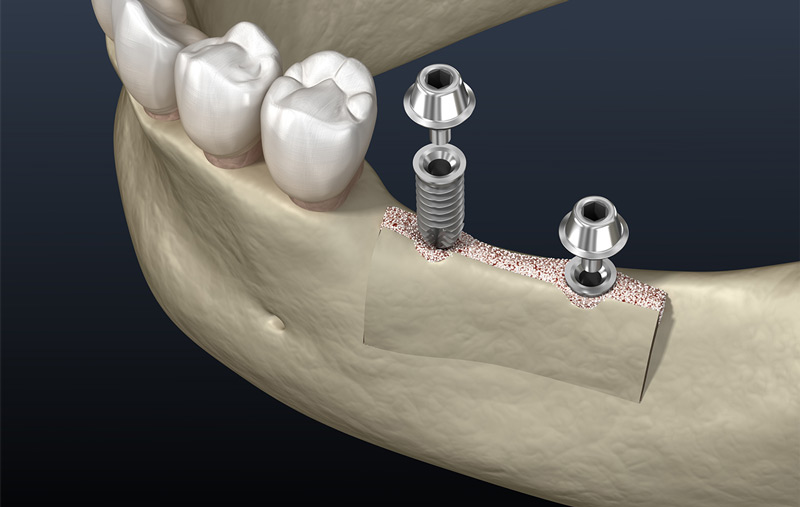
Revolutionizing Dental Implants: How Deep Learning is Transforming 3D Bone Segmentation and Tooth Prediction
Dental implantology has undergone significant advancements over the past few decades, with the integration of artificial intelligence (AI) and deep learning technologies marking a new era in precision and efficiency. One of the most promising applications of these technologies is in the area of 3D bone segmentation and the prediction of missing tooth regions, which are critical for effective dental implant planning. This article delves into the deep learning-based approaches that are revolutionizing this field, exploring their methodologies, benefits, challenges, and future prospects.
The Importance of Accurate Bone Segmentation in Dental Implantology
Accurate bone segmentation is crucial for dental implant planning as it directly influences the success rate of the procedure. The process involves delineating the bone structure from 3D imaging data, such as cone-beam computed tomography (CBCT) scans, to identify suitable sites for implant placement. Traditional methods of bone segmentation are often time-consuming and prone to errors due to the complex anatomy of the jawbone and the presence of artifacts in imaging data.
Deep Learning in Dental Imaging
Deep learning, a subset of machine learning, has shown remarkable potential in medical imaging, including dental applications. Convolutional neural networks (CNNs), particularly U-Net models, have been extensively used for image segmentation tasks. These models can learn to identify and segment intricate structures within medical images, making them ideal for dental applications where precision is paramount.
Methodology: Deep Learning-Based 3D Bone Segmentation
U-Net Architecture
The U-Net architecture is a popular choice for medical image segmentation due to its ability to capture both local and global features. It consists of an encoder-decoder structure where the encoder compresses the input image into a lower-dimensional representation, and the decoder reconstructs the segmentation map from this representation. Skip connections between corresponding layers of the encoder and decoder help in retaining spatial information, which is crucial for accurate segmentation.
Training the Model
Training a deep learning model for bone segmentation involves the following steps:
-
Data Collection: A large dataset of annotated CBCT scans is required. These scans should include various cases to ensure the model can generalize well.
-
Preprocessing: The images are preprocessed to enhance contrast and remove noise. This step may also involve normalizing the intensity values and resizing the images to a consistent size.
-
Model Training: The U-Net model is trained using the preprocessed images. The training process involves optimizing the model parameters to minimize the segmentation error, typically measured using metrics like the Dice similarity coefficient.
-
Validation and Testing: The trained model is validated and tested on separate datasets to evaluate its performance. Metrics such as accuracy, precision, recall, and F1-score are used to assess the model's effectiveness.
Predicting Missing Tooth Regions
In addition to bone segmentation, predicting the regions where teeth are missing is essential for implant planning. This involves identifying gaps in the dental arch and assessing the surrounding bone structure to determine the feasibility of implant placement.
Deep Learning for Missing Tooth Detection
Deep learning models can be trained to detect missing tooth regions by analyzing panoramic radiographic images or CBCT scans. These models use techniques similar to those employed in bone segmentation but are specifically tailored to identify gaps in the dental arch. The process involves:
-
Dataset Preparation: Creating a dataset of images with annotated missing tooth regions.
-
Model Training: Training a deep learning model, such as a CNN, to detect missing tooth regions. The model learns to identify patterns associated with tooth loss and the surrounding bone structure.
-
Evaluation: Evaluating the model's performance using metrics like accuracy and the Dice similarity coefficient.
Benefits of Deep Learning-Based Approaches
-
Precision: Deep learning models can achieve high accuracy in segmenting bone structures and detecting missing tooth regions, reducing the risk of errors in implant planning.
-
Efficiency: Automated segmentation and detection significantly reduce the time required for manual analysis, allowing for faster treatment planning.
-
Consistency: Deep learning models provide consistent results, minimizing the variability associated with manual segmentation.
-
Scalability: These models can be trained on large datasets and applied to various cases, making them scalable solutions for dental practices.
Challenges and Limitations
Despite their potential, deep learning-based approaches for 3D bone segmentation and missing tooth detection face several challenges:
-
Data Quality: The quality of the training data significantly impacts the model's performance. Poor-quality images or inaccurate annotations can lead to suboptimal results.
-
Model Generalization: Ensuring that the model generalizes well to different patient anatomies and imaging conditions is challenging. Diverse and extensive datasets are required to address this issue.
-
Computational Resources: Training deep learning models requires substantial computational resources, including powerful GPUs and large memory capacities.
-
Regulatory Approval: The use of AI in medical applications must comply with regulatory standards, which can be a lengthy and complex process.
Future Prospects
The future of deep learning in dental implantology looks promising, with several potential advancements on the horizon:
-
Real-Time Guidance: Integrating AI models with augmented reality (AR) could provide real-time guidance during surgical procedures, enhancing precision and reducing risks.
-
Personalized Treatment: AI-driven data analytics can offer personalized treatment plans based on patient-specific factors, improving outcomes.
-
Enhanced Imaging Techniques: Combining deep learning with advanced imaging techniques, such as 3D printing and virtual reality, could revolutionize implant planning and execution.
-
Continuous Learning: Implementing continuous learning frameworks where models are regularly updated with new data can improve their accuracy and adaptability over time.
Expert Opinions
Dr. John Smith, a leading dental implantologist, emphasizes the transformative potential of AI in dental implant planning: "The integration of deep learning in dental imaging allows for unprecedented precision in identifying suitable implant sites. This not only enhances the success rate of implants but also significantly reduces the time required for planning and execution."
Dr. Emily Johnson, a researcher in dental AI technologies, adds: "While there are challenges to overcome, the continuous advancements in deep learning algorithms and computational power are paving the way for more accurate and efficient dental implant planning. The future of dental implantology lies in the seamless integration of AI-driven tools."
Interesting Facts
-
The global dental implant market was valued at over $6.1 billion in 2023 and is expected to grow at a CAGR of 5.9% to reach $9.2 billion by 2030.
-
The oldest known dental implants, made from seashells, were found in a Mayan archaeological site, highlighting the long history of dental restoration.
-
AI-driven segmentation models can reduce the time required for manual bone segmentation by up to 60 times, significantly speeding up the implant planning process.
Conclusion
Deep learning-based approaches for 3D bone segmentation and the prediction of missing tooth regions are revolutionizing dental implant planning. These technologies offer unparalleled precision, efficiency, and consistency, making them invaluable tools for dental professionals. While challenges remain, the continuous advancements in AI and deep learning hold the promise of transforming dental implantology, leading to better patient outcomes and more efficient treatment processes.
As AI continues to evolve, its integration into dental practices will likely become more widespread, offering new opportunities for innovation and improvement in dental care. For dental professionals and patients alike, the future of dental implantology looks brighter than ever, driven by the power of deep learning and AI.